Understanding Supervised vs Unsupervised Learning A Beginner's Guide
- Gowtham V
- Nov 6, 2024
- 4 min read
Machine Learning (ML) is rapidly transforming industries enabling companies to make data driven decisions, automate processes and improve customer experiences. At the heart of machine learning there are two core approaches: supervised learning and unsupervised learning. Understanding the difference between these type of learning is crucial for anyone entering the field of AI and Data Science. In this beginner's guide, we'll dive into what each approach entails, how they work, and when to use them.
What is Machine Learning?
Machine Learning is a branch of Artificial Intelligence (AI) where computers learn from data to make predictions or decisions without being explicitly programmed. It allows systems to automatically improve and adapt based on experience. Machine Learning can be broadly divided into Supervised Learning, Unsupervised Learning and Reinforcement Learning. This guide will focus on the first two learning (Unsupervised and Supervised Learning), as they are fundamental to understand how ML models work.
Supervised Learning
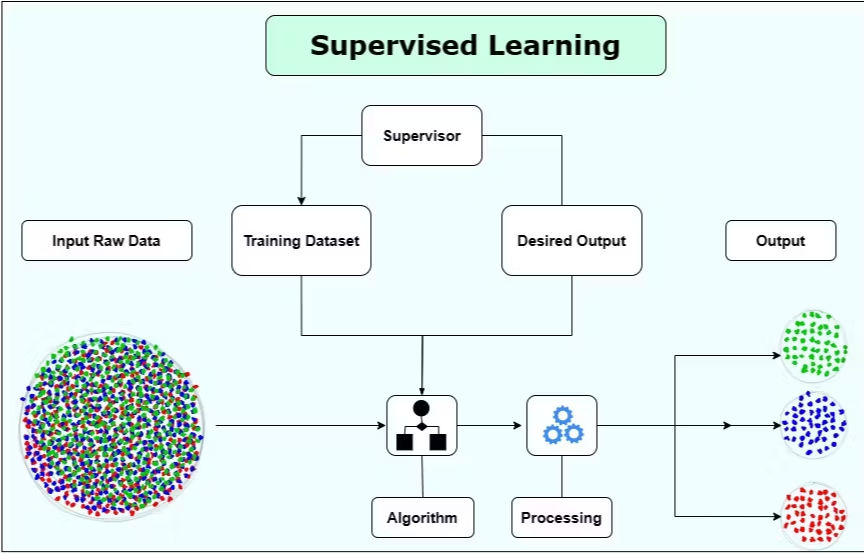
Supervised learning is the most commonly used type of machine learning. In supervised learning, algorithms are trained on a labelled dataset, which means that each input is paired with correct output. The model learns from these examples to make predictions or classifications for new data.
How it works?
Imagine you are training a model to predict house prices. You would start with a dataset where each house has features (size, location and number of bedrooms) and a corresponding price. After training, the model can predict prices for new houses based on learned patterns.
Common Algorithms in Supervised Learning
Regression: Linear Regression, used for predicting continuous values like house prices.
Classification: Logistic Regression, Decision Trees and Support Vector Machines (SVM), used for categorizing data (e.g., spam vs. non-spam emails).
Applications:
Email Spam Detection: Classifying emails as spam or not spam based on labelled examples.
Image Classification: Identifying objects in images like detecting dogs or cats.
Sentiment Analysis: Identifying social media posts to determine if the sentiment is positive, negative or neutral.
Unsupervised Learning:

Unlike supervised learning, unsupervised learning works with unlabeled data. This means that this algorithm doesn't have predefined outcomes; instead it finds hidden patterns or groupings within the data. Unsupervised learning is often used for exploratory data analysis, where we want to uncover natural groupings or detect anomalies.
How it works?
Let's say we want to segment customers based on purchasing behaviors. With unsupervised learning, we'd input data based on customer purchases, and the algorithm would identify clusters of similar clusters without knowing who belongs to which segment. This can reveal valuable insights into customer preferences.
Common Algorithms in Unsupervised Learning:
Clustering: K-means and Hierarchical Clustering used for grouping data points into clusters.
Association: Apriori Algorithm used in market based analysis to identify product associations.
Dimensionality Reduction: Principal Component Analysis (PCA) is used to reduce the complexity of the data.
Applications:
Customer Segmentation: Grouping customers into segments for targeted marketing.
Anomaly Detection: Identifying unusual data points, useful for fraud detection.
Recommendation Systems: Suggesting products to customers based on similar preferences.
Key Differences between Supervised and Unsupervised Learning:
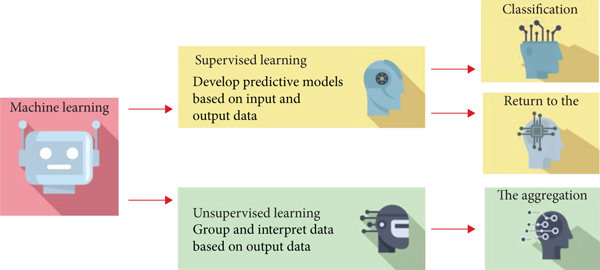
To clarify the differences between these types of learning here's a quick comparison:
Aspect | Supervised Learning | Unsupervised Learning |
Data Type | Labeled (input-output pairs) | Unlabeled (only input data) |
Goal | Predict or Classify Outputs | Discover Patterns or Groupings |
Common Algorithms | Regression, Classification | Clustering, Association |
Applications | Email spam detection, image classification | Customer Segmentation, Anomaly Detection |
Supervised learning is ideal for cases where labeled data is available and we want to make predictions. Unsupervised learning on the other hand, is useful for exploring datasets to uncover hidden structures.
When to use Supervised vs Unsupervised Learning ?
Knowing when to apply each type of learning is essential
Use Supervised Learning: When you have labeled data and need a model that can predict outcomes. For example, predicting customer churn based on past behavior or classifying diseases based on patient symptoms.
Use Unsupervised Learning: When you are working with unlabeled data and want to explore patterns, like grouping customers based on purchase history or detecting fraudulent transactions.
Real World Examples:
Example of Supervised Learning:
Email Spam Detection: In this task, a supervised learning algorithm is trained on a dataset of emails labeled as "spam" or "not spam". The model learns to recognize patterns in spam emails and can then classify new emails based on this knowledge.
Example Of Unsupervised Learning:
Customer Segmentation: For a retail company, unsupervised learning can help segment customers into groups based on purchasing habits. By identifying these groups, the company can create personalized marketing campaigns for each segment.
Conclusion:
Both supervised and unsupervised learning are powerful techniques in the world of machine learning, each suited to different types of problems. Supervised learning is perfect when you have labeled data and a clear outcome in mind, while unsupervised learning is ideal for discovering patterns and structures within unlabeled data.
By understanding these differences, you'll be well-prepared to choose the right approach for various machine learning tasks. Whether you're a beginner or looking to deepen your knowledge, experimenting these types of learning is a great way to strengthen your skills in AI and ML.
Ready to dive deeper? Start experimenting with datasets and try applying both supervised and unsupervised algorithms to see how they work in real world scenarios.
Comments